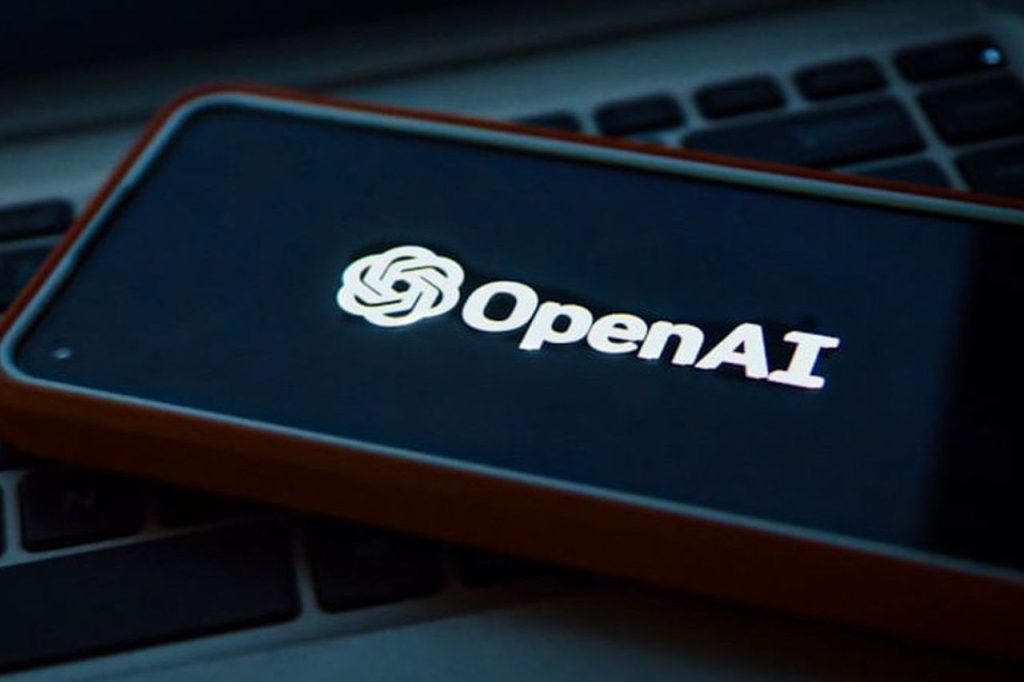
When OpenAI first revealed its o3 Ai model back in December, it was a big development. The company proudly stated that o3 could correctly answer over 25% of questions on FrontierMath, a infamously difficult set of math problems used to gauge advanced reasoning in AI systems. At the time, this looked like a major jump forward no other model had scored higher than 2% on the benchmark.
Mark Chen, OpenAI’s chief research officer, even emphasized during a livestream that all offerings out there have less than 2% on FrontierMath and that OpenAI’s internal tests using high compute settings pushed o3 beyond the 25% mark.
However, recent independent testing tells a different story.
Epoch AI, the organization behind the FrontierMath benchmark ran its own evaluation of o3 after the model’s public release in April 2025. Their results? Around 10% accuracy, a noticeable drop from OpenAI’s touted peak performance.
So what’s going on here?
Well, the answer isn’t so much about deception as it is about context. The version of o3 that OpenAI publicly launched appears to be different and less powerful than the one used in its December demonstrations. According to Epoch, several factors could explain the performance gap: differences in compute resources, the specific subset of problems tested, or changes in the benchmark dataset itself.
A post from the ARC Prize Foundation, which had early access to a preview version of o3, backed this up. They confirmed that the o3 tested earlier was not the same as the current public release, describing it as a more capable system likely operating with higher compute tiers. Those bigger models naturally tend to perform better but are costlier and slower and less suited to real-world deployment.
Even OpenAI’s own technical staff acknowledged this. Wenda Zhou, speaking on a recent livestream, noted that the released version of o3 had been fine-tuned for faster, more cost-efficient use not necessarily for acing benchmarks. In other words, OpenAI made trade-offs to optimize the model for real-world applications like chat and coding, rather than squeezing every drop of performance out of it in ideal conditions.
Interestingly, OpenAI’s newer models o3-mini-high and o4-mini are reportedly outperforming the original o3 on FrontierMath, showing that the company is still pushing the envelope, even if benchmark scores fluctuate.
This isn’t the first time a benchmark drama has hit the AI world. Meta and Elon Musk’s xAI have both been called out recently for inflating benchmark results or being less than clear about the models tested. And back in January, Epoch itself took some heat for failing to disclose OpenAI’s funding until after the original o3 announcement, stirring concerns about transparency.
Related links you may find interesting
The Bigger Picture
While these debates may seem like technical squabbles, they reflect a deeper challenge in the AI space: How do we meaningfully evaluate models when performance can vary so wildly depending on the context and the company behind the data?
Benchmarks like FrontierMath, MMLU, and ARC-AGI provide helpful metrics, but they’re not the full story. What really matters for users whether developers, businesses, or the general public is how these models perform in real-world settings.
OpenAI is reportedly planning to release o3-pro soon, a more powerful tier expected to be closer to that original high-performance version. If that happens, we might see benchmark scores rise again along with a new wave of comparison debates.
When it comes to OpenAI o3 Ai model performance, context is everything and benchmarks should be read with a healthy dose of curiosity and caution.